With AI and generative AI increasingly at the center of many digital transformation initiatives, data is also core to success with these technologies. So, how can big ag leaders make sure they have the right approach in place to ensure their data is ready for success?
A Data-First Strategy for Big Ag Digital Transformation
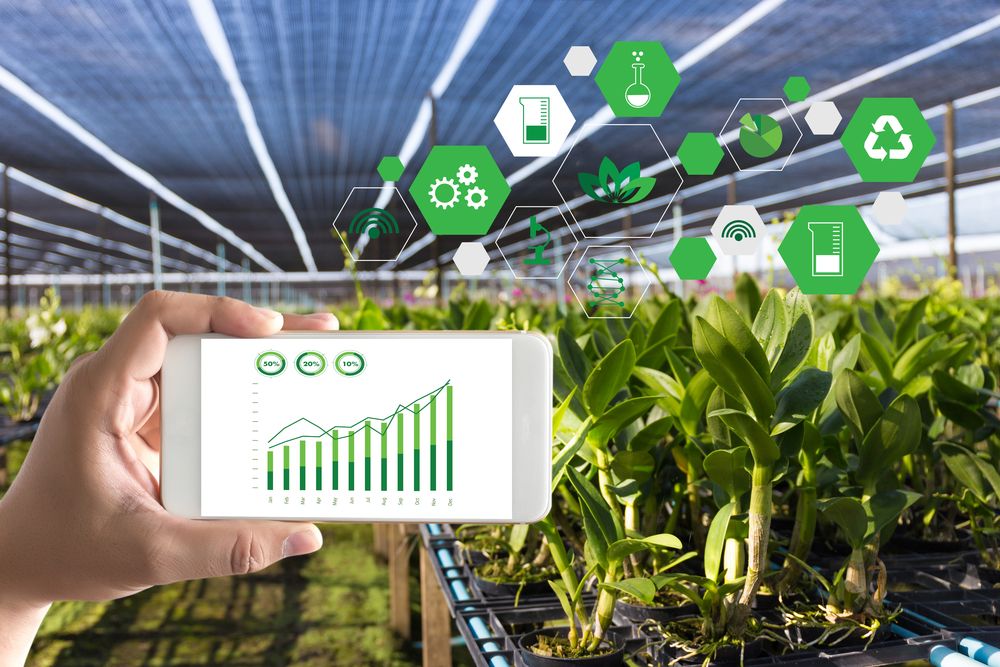
Brian Regan, senior vice president, Americas delivery | Syniti
Digital transformation offers huge potential for operational efficiency gains in the agricultural sector, but any return on investment requires high-quality data. Without the right data foundation, no digital transformation effort will be truly successful. Ensuring the right data foundation to achieve results requires implementing a strategic approach to data that can scale as a company grows and evolves.
With AI and generative AI increasingly at the center of many digital transformation initiatives, data is also core to success with these technologies.
So, how can big ag leaders make sure they have the right approach in place to ensure their data is ready for success? The key is to put data first.
What does it mean to be data-first?
Over the last 20 years, many large agriculture companies have invested significantly in different types of system transformation initiatives, whether implementing ERP, CRM or PLM systems. For companies in this space, it tends to be all about scale and process efficiency, and their transformation investments reflect that.
For instance, let’s say you’re a commodity company that sells soybeans to a customer who also does business with your competitor. You win by ultimately making your beans more affordable for your customer, which you can do by having the most efficient and capable transportation management system to drive down the cost of getting the beans to the customer. All that means companies in this space prioritize efficiency, but ensuring the success of the systems they adopt to be more efficient depends upon the quality and accuracy of the data within those systems. This is true whether it’s implementing a new CRM system to gain better visibility and understanding of customers, implementing ERP systems to better manage materials and inventory and planning across their organization, or any other similar initiative. As companies grow and have operations in multiple locations, combining disparate data from those locations into a single system becomes a bigger challenge. However, consolidating, deduplicating and cleaning the data before it goes into the systems absolutely must be the first step, regardless of how much of an uphill battle it might seem. Without the right data in the systems, these transformation initiatives will fail. That is the bottom line.
Why hasn’t data-first always been the case?
A data-first mindset is not the default approach because it is hard. Data is intrinsically cross-functional. If we go back to the soybean example above, you have to understand how much it costs your company to grow them or purchase them from a grower. You must understand how long it takes to transport them to customers in different locations. Increasingly, companies must also be able to provide information like where those soybeans originated from before they’re shipped all over as aspects like traceability become more critical.
Accurate answers to all of these questions require accurate input from various distributed parts of the organization, and that’s difficult.
What has changed is that failure is not an option like it used to be. In addition, regulations are evolving, and customer demands are changing. Previously, you could spend money on a data warehouse initiative or produce metrics no one trusted. At the same time, you could pick, pack, ship, sell and report to the street; you could run your business relatively well. Given the competitive environment that exists now, it's not an option for large enterprises to have poor-quality data, suboptimal systems, excess inventory, and poor planning parameters – these all become much more painful for organizations. Inefficiency can quickly lead to your customer buying more corn from the competition.
Change management for a data-first approach
The data-first approach requires change management. For this, you need a clear eye on the business benefits you’re trying to secure through your transformation initiatives and an objective appraisal of what you’ll need to secure those benefits.
When you spin up a large program in a corporate environment, there's always the initial tendency or temptation for excess enthusiasm. You hear what the analysts and salespeople say about the new system you're about to implement, and you talk to other customers who mention how outstanding their implementation was and all the benefits they secured.
In those moments, it's easy to forget about what you’ll need to do to achieve similar levels of success. When discussing change management, stay clearly focused on the business benefits and consider what is required from a data perspective to secure them.
The top three advantages to taking a data-first strategy
There are three data-first advantages to strive for. The first is securing the foundational conditions to glean business benefits. You need accurate customer data if you want a 360-degree understanding of your customers. If you want to understand your spend, you need accurate supplier information. If you want to optimize your inventory, you need accurate parts information. So, fundamentally, you need accurate, high-quality information in your systems to improve business performance.
The second advantage is the implementation cost itself. Modern transformation initiatives are expensive. Usually, it’s an 8- to 10-figure outlay. What’s more, data is usually one of the leading causes of delays. If you're burning five or tens of millions of dollars a month, a three-month delay gets very expensive. Look at the overall investment associated with implementing digital transformation initiatives. You can save quite a bit of money by getting data right, whether by accelerating programs or mitigating the risk of delay.
The third is risk avoidance. If inaccurate data informs your business decisions, that can lead to adverse outcomes. From an operational risk perspective, you will tend to see benefits if you adopt a data-first strategy.
Commit to the data first
Do not miss the opportunity to get data right. If you’re investing 8 to 10 figures on a digital transformation initiative, the worst mistake you can make is to put the wrong data into that system. That will inhibit you from making good business decisions for the next decade.
Yes, a data-first approach can be difficult – but years of skewed “insights” and poor business decisions can lead to the even harder reality of lost profit, increased costs or missed revenue opportunities. A well-designed data-first initiative can drive internal efficiencies by virtue of digital transformation. Stay optimistic but realistic and keep your eyes on the prize: a system that yields accurate insights and helps secure your business’s future.
Brian Regan is the SVP, Americas Delivery for Syniti, located in Needham, Massachusetts. Brian is responsible for managing customer partnership and client-facing delivery success. Brian drives key executive relationships and is responsible for growing successful partnership at Syniti’s client portfolio.
Brian returned to Syniti through the acquisition Data Migration Resources in 2021. As the SVP, Go-To-Market at DMR, Brian was responsible for strategic relationships and customer growth as the company expanded from a niche $5M consultancy to a $40M global company.
The content & opinions in this article are the author’s and do not necessarily represent the views of AgriTechTomorrow
Comments (0)
This post does not have any comments. Be the first to leave a comment below.
Featured Product
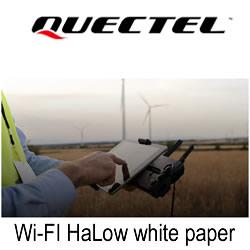