Hopefully, as confidence grows in our ability to forecast weather and soil conditions, much value will be extracted for more efficient operations, higher yields, and better environmental stewardship.
Brent Shaw | Iteris
Do you ever wonder exactly how the weather forecast is made? And how good are they, really?
In last month’s article, I discussed the accuracy and usefulness of seasonal weather outlooks. This month, I want to hone in on near-term weather forecasts, defined for our purposes as forecasts of specific day-by-day or even hourly conditions for periods less than two weeks into the future.
These are the forecasts by which we plan our weather-sensitive operations and personal activities. This is my career passion because it is in these time frames that confidence is high enough to make significant decisions that directly affect agricultural operations and output. Yet, my observation is that weather forecasts are still under-utilized in agricultural decision-making processes, with an over-emphasis on using recent and past data for determining future actions, perhaps due to the historical perception that forecasts are no better than the flip of a coin.
Hopefully, this article can help build confidence in modern weather forecasting capabilities.
What Makes a Forecast?
The weather forecasting process starts with the collection, quality control, and fusion of large amounts of diverse raw observational data from space, airborne, and ground-based assets to produce a coherent, three-dimensional view of the atmosphere at any point in time, from which we project the future conditions, adjust, and repeat. Those are all large topics for another time. For now, let’s just focus on the actual methods used in projecting the weather forward in time from the current conditions.
It turns out there are multiple techniques that are used, each of which has specific time frames for which they are most relevant. These methods are shown in the figure below, which depicts their comparative usefulness as a function of forecast lead time (meaning, how far into the future are you trying to predict, e.g., a forecast for tomorrow would have a lead time of one day).
The two simplest methods are persistence and climatology.
A persistence forecast means that conditions are assumed to remain unchanged. We all do this inherently when we look out the window to see if an umbrella or sunglasses are needed for an immediate, short-lived outside activity because in most cases we can assume things remain constant for a few minutes or longer.
A climatology-based forecast assumes conditions for a particular day or time period will fall within statistical normals derived over many years for that same day or period. Interestingly, these two “easy” methods are applicable at opposite ends of the time spectrum, with persistence often working very well for forecasting very near term conditions, and climatology being the best predictor for long-range forecasts. For a forecast to be considered to have any skill, it must be able to at least beat both of these two methods.
Extrapolation is slightly more complicated, and assumes an existing weather feature, such as a cold front or a line of storms, will continue generally moving in the same speed and direction without significant change. But it cannot account for new development or dissipation. We will save teleconnections for another time, as it is primarily for long-range forecasting, using large scale indicators such as the El Niño Southern Oscillation to infer what might be expected for the longer-range patterns.
That leaves numerical weather prediction, which is by far the most important method used in modern meteorology for forecasting lead times beyond a couple of hours. The advent of affordable, high-performance computing has revolutionized our ability to create computer models that simulate the motion and physics of the three-dimensional atmosphere, accounting for its interaction with topography, land surface, and the oceans with amazing realism, without any need for historical data or statistical relationships.
You often hear the TV meteorologist refer to “the models” when talking about the forecast, often accompanied with realistic animations of future clouds and precipitation. In the last few decades, the advancement of these models have dramatically improved forecast accuracy and changed the role of the human weather forecaster. Yet, they still are subject to errors due to our incomplete understanding of all process interactions, our inability to explicitly simulate down to chaotic, molecular levels, and insufficient sampling of the initial state of the atmosphere, particularly in the mid and upper levels of the atmosphere over the oceans and other unpopulated areas. Thus, in most cases, the best weather forecast is an optimum blend of some or all of these methods, most often performed by a combination of automation and human oversight.
How Accurate Is It?
So, how good are the near-term forecasts now? ForecastWatch.com is an independent weather forecast verification service. Each day they collect and archive daily forecasts from a large number of public and private weather forecast providers. They compare these forecasts to weather observations from high-quality, government-operated weather stations and generate statistics on a variety of weather parameters of interest to the general public, including daily high and low temperature, wind speed, and occurrence of precipitation. This provides an excellent “apples-to-apples” comparison across providers because the same methods and truth data are applied to all sources, and the consistency allows for the assessment of long-term changes in accuracy. They recently published their study of daily high-temperature forecast accuracy, based on a 12-year period spanning 2005 through 2016, aggregating almost 200 million individual data sample for over 750 U.S. locations. If you like numbers and graphs, it’s worth a read. But, some key takeaways are summarized here:
- One-day forecasts have an error of less than 3° Fahrenheit.
- The five-day forecast is now almost as accurate as a one day forecast was in 2005, and overall error of the daily high-temperature forecast decreased by 33% over that period.
- We have now reached a point where the nine-day temperature forecast is slightly better than climatology.
The graph below shows statistics from ForecastWatch for this year, spanning January through September. It shows the forecast error in degrees by forecast lead time for a climatology-based forecast (black), persistence forecast (red), and the average error of all providers analyzed by their service (blue). As mentioned, a forecast is really only useful if it performs better than persistence and climatology, and you can see that is the case all the way through day nine. This is consistent with the conclusions drawn from the 12-year study. The error tends to increase roughly 0.5° per day, so you can also infer that by around 10 days out, the forecasts are no longer more accurate than climatology, corroborating my assertion in last month’s article that forecasts of specific conditions at a particular location beyond a couple of weeks are beyond the state of the science.
As models continue to improve, we can expect to see a continuation of improving forecast accuracy, at least to a point. There is much debate about what the realistic limits are for weather forecasting, and it may not ever reach a point where we can project exact weather for a location beyond a few weeks in our lifetimes. But, in terms of things we attempt to predict in this world, the forecasting of near term weather is a major success story.
Hopefully, as confidence grows in our ability to forecast weather and soil conditions, much value will be extracted for more efficient operations, higher yields, and better environmental stewardship.
The content & opinions in this article are the author’s and do not necessarily represent the views of AgriTechTomorrow
Comments (0)
This post does not have any comments. Be the first to leave a comment below.
Featured Product
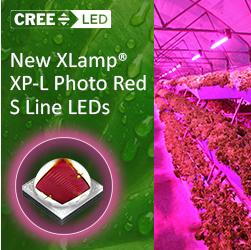