Its the underlying science tools and heroes of the digital agriculture movement - specifically, machine learning and predictive modeling - that are central to the success of delivering a digital transformation.
Bringing Silicon Valley to the Farm
Sam Eathington | The Climate Corporation
Reprinted with permission from The Climate Corporation blog:
In January 2017, we became the first digital agriculture company to share our innovation pipeline publicly - favoring transparency and access over closed-door science. This January, we announced 10 additional advancements for a total of 17 advancements over the last year. We believe this openness is powerful for our customers and momentum-building for the industry. It also invites a deeper discussion around how new data innovations are transforming agriculture.
Looking back over the last decade, it’s incredible to reflect on the shift taking place in agriculture. In a precious few years, we’ve crossed a threshold into an age in which the specificity of industry agronomic data is no longer broad, regional, or generic - it’s personalized to individual fields. For the first time in the 10,000-year history of agriculture, macro data streams can be seamlessly intertwined with micro, field-level data to provide insights and a new level of value for farmers.
More simply put, Silicon Valley has moved to the Midwest and is at the farmgate.
Agriculture's Leading Digital Innovation Pipeline
As the leader bringing farmers around the world increased productivity through digital agriculture tools, our team at The Climate Corporation continues to push the envelope. For us, it’s all about delivering more value through easy-to-use tools with the Climate FieldView™ platform. For FieldView™ customers, you’ve already experienced this while assessing product performance with yield analysis or using advanced seed scripting. In 2017, Farmers using advanced seed scripting saw an average increase of 5 bu/ac compared to users who wrote their own scripts.*
Stepping back and looking at our dozens of projects and advanced data applications in development - analyzing all the variables that impact yield - I can tell you, with confidence, that the best is yet to come.
The Science Powering Digital Agriculture
It’s the underlying science tools and heroes of the digital agriculture movement - specifically, machine learning and predictive modeling - that are central to the success of delivering a digital transformation. To make these strides possible, our teams, made up of leading experts in multiple fields, are writing the book (or code) on delivering solutions unique to our customers’ fields.
Machine learning is a critical component of almost every science project we drive forward. By “training” tech tools to be on the look-out for certain field issues, we can help farmers take action before yield-robbing events occur. Like machine learning, our use of predictive modeling also runs on massive volumes of data. The key difference is we’re not looking for a “smart” computer decision or alert, we’re looking for a specific agronomic answer. What results in a simple recommendation for a field is powered by an incredibly complex combination of formulas and data streams. From an innovation and design standpoint, it’s beautiful. For our customers, it’s simple, personalized, and valuable. Here are some examples of how these capabilities span our entire innovation pipeline:
Disease Management: Through machine learning, we’re able to categorize the color, size, and scale of lesions on an individual plant and make a disease diagnosis. Think when Facebook or Google automatically tags a photo - only for your crops instead of your friends and family.
Seed Scripting and Selection: In our seeding research, we analyze field variability and bring this information together with data from numerous sources - like seed genetics, traits, imagery, disease pressure, soil composition, water movement, weather, and decades of historical performance data - to advance our machine learning and predictive models which help farmers understand how to select the right seed in the best place for maximum performance.
Nitrogen and Fertility Management: We embrace the complexity of soil fertility cycles using advanced modeling. For example, with nitrogen, this allows us to capture key processes including volatilization, denitrification, leaching, and plant uptake. We’re applying a similar approach with other key nutrients as we work to build a comprehensive fertility solution for farmers, including phosphorus and potassium scripting for new crops and new geographies.
Now that Silicon Valley sensibilities have taken root in the Midwest, the possibilities for ag’s digital revolution are immense. For us, it’s full steam ahead leading the digital agriculture charge. You can count on us to keep pushing science tools and innovation forward, unlocking new insights and customer value throughout the season.
*Assumptions:
- Comparing 281,000 ac in 4,179 fields
- More than 25% of field had script
- Products maturity ranges between 95-115 range
- Limiting comparisons to the same county; represents 79 counties 10 Midwest states
- Minimum of 3 Advanced & DIY Seed scripts in a county to be compared
The content & opinions in this article are the author’s and do not necessarily represent the views of AgriTechTomorrow
Comments (0)
This post does not have any comments. Be the first to leave a comment below.
Featured Product
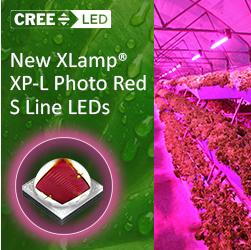