This data will be used to improve our understanding of ongoing trends in crop nutrient uptake and removal and make it easier to create decision support systems to determine how to optimize crop production in a sustainable way under changing environmental conditions.
Wageningen University, IFA, and Agmatix Collaborate in an Ongoing Project to Analyse Crop Nutrients
Case Study from | Agmatix
Crop nutrient optimization across multiple production environments requires large amounts of high-quality data. In an innovative research project, Wageningen University (WUR) and the International Fertilizer Association (IFA) are collecting data from multiple researchers and institutes to build a first-of-its-kind database of nutrients in crops under an array of environmental conditions. This database will be open to all data contributors seeking to support their research and will be made available to the general public (once the publication has been accepted). This data will be used to improve our understanding of ongoing trends in crop nutrient uptake and removal and make it easier to create decision support systems to determine how to optimize crop production in a sustainable way under changing environmental conditions.
To date, more than 30 researchers have shared data from 50 countries about four nutritionally and industrially important crops–maize, rice, soybeans, and wheat. Unfortunately, their data files differed considerably in terms of structure and parameter nomenclature. Thus, it will be extremely challenging to analyse them in a meaningful way to obtain insights—if at all.
We will focus on a subset of data about a single nutrient: nitrogen. This nutrient is particularly important for corn production. However, it poses an interesting dilemma: Large quantities of nitrogen are needed to ensure high yields of corn, but too much nitrogen can be detrimental to the environment—leaching from the soil to pollute waterways and contribute to greenhouse gas emissions. There is a need to better understand nutrient budgets in production environments, to allow for optimization of fertilizer applications, and to manage production in a more sustainable way. This can be achieved by developing a model to predict site-specific crop nitrogen removal rates.
Challenge
To develop a better understanding of the dynamics of corn nitrogen uptake and the factors affecting it, one needs to collect data from multiple nitrogen-producing environments so they can be compared and analysed. The fragmentation of the data, and the lack of common naming and protocols, hampers our ability to generate such a unified dataset.
Solution
To address this challenge, WUR and IFA teamed up with Agmatix. Agmatix is an AgTech start-up that has developed a state-of-the-art technology platform that transforms big data into powerful models. Using AI technology and an in-house ontology protocol (GUARDS -Global Universal Agronomic Data Standard), the platform can be used to automatically standardize and harmonize agronomic data from everywhere and anywhere into a unified format. Once standardized, data can provide insights that can be used to generate a variety of statistical models and predictions.
Results
Data of 5,377 observations collected from three countries – the United States, China, and Nigeria were standardized and harmonized using the Agmatix platform. Multiple covariates such as nutrients input, soil texture, organic matter in the soil, and cultivar maturity were augmented with site-specific weekly rainfall and temperature data. These data served as the basis for a machine learning model to generate an ensemble of decision trees to predict grain nitrogen concentration. 25% of the data were not used for calibration and were used for validation. The model was used successfully to predict the percentage of nitrogen in grain using data from these three countries (Figure 1), with a mean absolute deviation (MAD) of 0.09 [% Nitrogen], and an average prediction error of 7.2%. A feature importance analysis found that cultivar maturity, nitrogen input, and soil organic matter had the greatest influence on nitrogen availability. Future work can extend the model to include more countries and production environments.
Results were taken from three key corn producing countries – the USA, China and Nigeria and showed that cultivar maturity, nitrogen input, and soil organic matter had the greatest influence on nitrogen availability.
Conclusion
The unique dataset collected by WUR and IFA, and standardized using the Agmatix platform, can be used to generate multiple insights and models to help optimize production across environments. The data collection is ongoing, and researchers and organizations are invited to join this initiative and contribute data towards this project. Further conclusions are expected over the coming years.
The unique dataset collected by WUR and IFA, and standardized using the Agmatix platform, can be used to generate multiple insights and models to help optimize production across environments.
The content & opinions in this article are the author’s and do not necessarily represent the views of AgriTechTomorrow
Comments (0)
This post does not have any comments. Be the first to leave a comment below.
Featured Product
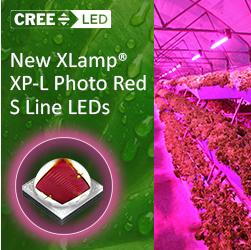