How AI Is Used for Crop Disease Diagnosis
AI is forcing agriculture workers to undergo digital transformation. The advantages the technology promises are too significant to ignore. With the climate more volatile than ever, yields are inconsistent and business resilience is unstable. Incorporating AI is one of the most effective ways to tackle a top farming fear — crop disease. How is the tech capable of accurately diagnosing sick crops?
Traditional Methods vs. AI in Crop Disease Diagnosis
Conventional farming techniques are time-consuming and inconsistently effective. Farmers had to inspect plants and do soil tests manually. The results varied if they misunderstood what they saw or had an inadequate sample size from the wrong location. Hands-on strategies served the agricultural industry for ages, but the frequency of human error meant they had to be phased out.
AI crop disease diagnosis provides comprehensive oversight more frequently and accurately. It leverages multiple technologies, such as predictive analytics, neural networks, computer vision and machine learning to familiarize farmers with the harvests as much as possible. Agricultural workforces will have unprecedented knowledge about their land’s wellness.
Efficacy is high in numerous testing environments. AI could detect leaf mold, spider mites, verticillium wilt, powdery mildew and more, with effectiveness ranging between 87% and 100%.
Applications of AI in Crop Disease Diagnosis
Digging into the nitty-gritty of AI’s applications reveals how versatile it is when identifying crop disease. AI collects information about crops, water and soil through sensors and other equipment. Machine learning systems use this database to make determinations.
AI uses incoming data and compares it against historical information about the land, crop species and known diseases to perform risk assessments. Once it determines a risk profile for each crop type based on the farmer’s unique land makeup, it may suggest prevention measures and solutions influenced by the most likely concerns.
Computer vision is another aid for informing these recommendations. AI pairs with cameras to spot visual differences in the plants. It is more likely to be the first to notice infectious bugs, differences in coloration and growth cycle changes by judging real-time images against pictures of healthy plants. If it detects anomalies, it will alert farmers.
Soil and water sources may also include sensors to feed into the AI’s datasets. They constantly test key performance indicators like pH, microbial concentrations and moisture. It may also detect the presence of foreign contaminants leading to disease.
Benefits and Challenges of Using AI for Disease Diagnosis
While the benefits outweigh the cons, potential obstacles are essential to acknowledge. This way, agriculturalists may prepare accordingly if their technology is ineffective. A contingency plan for AI malfunctions is crucial to securing a bountiful harvest and nourishing the planet.
The first hurdle is being patient with the high learning curve in the beginning. Many farmers are undergoing tech installations for the first time in their land’s history. During the adjustment period, workers may make mistakes or skew analytics. While this is normal, weeding out inaccuracies as early as possible is critical for data integrity. Lingering errors cause staff to misinterpret information and miss disease diagnoses altogether.
Additionally, some regions need to be more suitable for widespread tech adoption. Critical infrastructure may not exist in rural areas. People in agriculture must advocate for basic connectivity to allow farmers in all areas to experience the boons of modern devices.
The advantages are significant if farms overcome the barriers. Analytics are more accurate than manual operations. Therefore, workers will be faster at identifying problems and preventing blights from spreading. It prevents revenue losses while boosting foundational stability and yield optimization, making agriculture more sustainable in the long term. The industry will save money and time on labor and disease recovery because AI tools are more adept at catching signs than manual work.
Exploring Successful Case Studies
PlantVillage is a project sponsored by Penn State University that assists farmers with AI integrations. Higher education institutions are essential in developing AI disease detection technologies as much as private businesses and startups. Around 22.6% of undergraduate students struggle with food insecurity, meaning forging solutions to this problem impacts more than farmers.
The university project installed its technology on a Nepali banana farm to combat pests and soil-borne pathogens causing disease. The situation had become so dire that these struggling farms were spending the equivalent of hundreds of dollars to stay afloat. The AI successfully identified many diseases impacting yield while discovering farming management practices compounding the problem.
AgriPredict is another groundbreaking women-led startup technology using AI to help heal plants. Most of Zambia’s outfits are smallholder, subsistence farms, so any sight of crop disease could be catastrophic. It identifies crop diseases, changes in weather and sales avenues. It simplifies AI by making it accessible through a smartphone app. It prevented a crop disaster from unfolding in 2016 and 2017, likely due to its ease of use and effectiveness.
Promising Plant Health With Tech
Machine learning, AI and related tools are instigating a revolution in plant care. Modern agriculture necessitates data-driven insights to meet the care needs of an ever-demanding farm. Protecting one plot could save countless others from receiving the same crop diseases. Investing in AI disease detection boosts revenue while safeguarding the rest of the planet.
Comments (0)
This post does not have any comments. Be the first to leave a comment below.
Featured Product
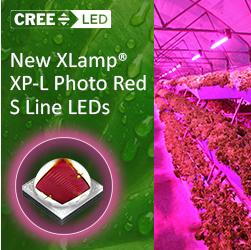